In the current digital age, industries like healthcare, pharmaceuticals, and education are sitting on a goldmine of untapped data — but are they making the most of it? Enterprise Resource Planning (ERP) systems, the backbone of organizational operations, collect vast amounts of data daily. However, many healthcare organizations struggle to extract meaningful insights from this data.
This is where Data Science comes into play. By integrating data science with ERP systems, healthcare providers can unlock hidden insights, optimize operations, and make data-driven decisions 🔬.
In this blog, we’ll explore how Data Science in Healthcare ERP Systems can revolutionize operations, drive innovation, and provide actionable insights. Whether you’re a healthcare provider, pharmaceutical company, or educational institution, this guide will help you leverage your ERP data for better outcomes ✨.
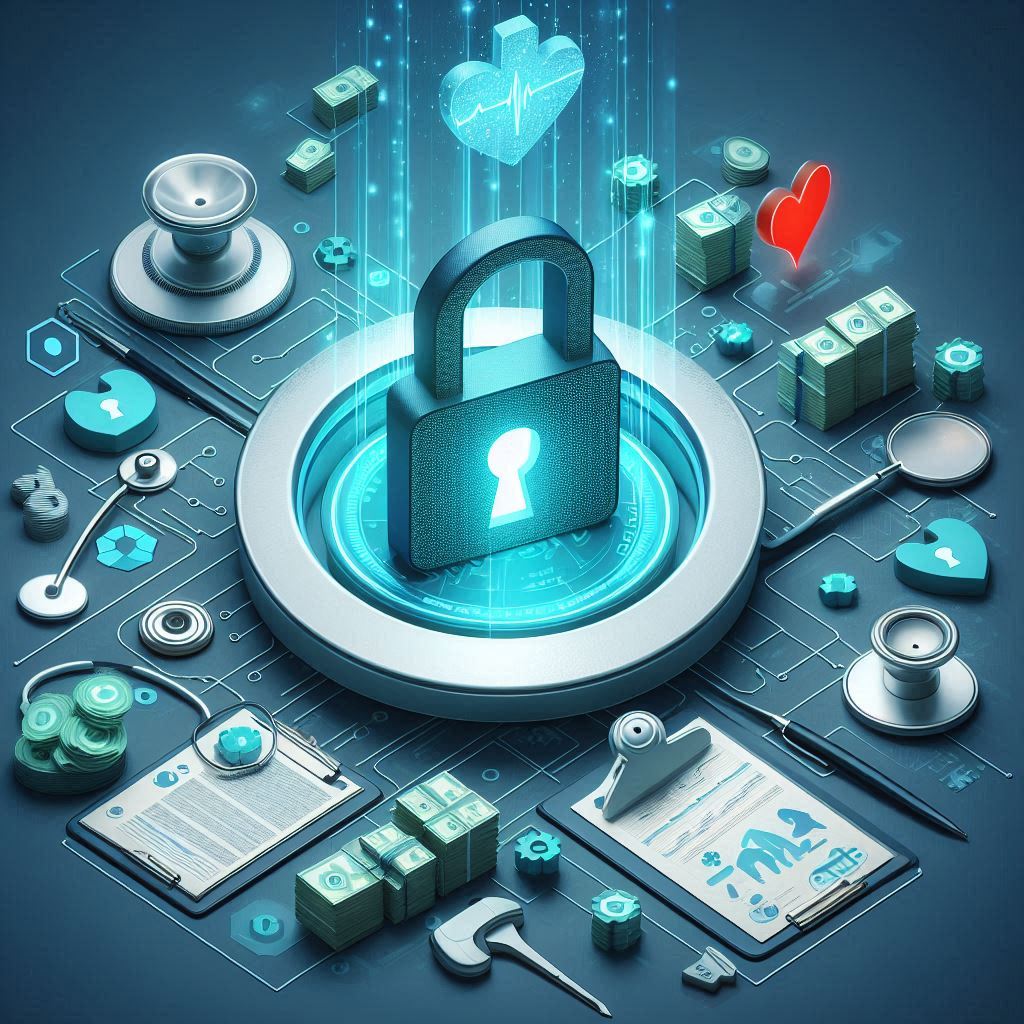
👨⚕️ Why Healthcare Needs Data Science in ERP Systems
The healthcare industry is one of the most data-intensive sectors, generating massive amounts of information from:
- Patient records
- Medical devices
- Supply chains
- Financial systems
Traditional ERP systems manage operations efficiently but often lack advanced analytics capabilities to derive actionable insights. Here’s why integrating Data Science in Healthcare ERP Systems is a game-changer:
- Improved Patient Outcomes — Analyze patient data to identify trends, predict diseases, and personalize treatment plans.
- Operational Efficiency — Optimize resource allocation, reduce wait times, and streamline supply chain management.
- Cost Reduction — Predictive analytics can identify cost-saving opportunities, such as reducing medication waste or optimizing staff schedules.
- Regulatory Compliance — Advanced analytics can ensure compliance with healthcare regulations by identifying anomalies and risks in real time.
🔍 What is a Data Science Proof of Concept (PoC)?
A Proof of Concept (PoC) is a small-scale project designed to test the feasibility of a new idea or technology. In the context of ERP systems, a Data Science PoC uses data science techniques to analyze ERP data and demonstrate the potential value of a full-scale implementation.
🎯 Key Objectives of a Data Science PoC:
- Validate Feasibility — Test whether data science can deliver actionable insights from ERP data.
- Demonstrate Value — Showcase potential benefits like cost savings, efficiency, and better decision-making.
- Identify Challenges — Highlight technical or operational roadblocks that need to be addressed.
- Build Stakeholder Buy-In — Use the PoC to convince decision-makers about the value of a full-scale implementation.
🔬 Steps to Build a Data Science PoC for Healthcare ERP Systems
A Data Science PoC for Healthcare ERP Systems involves multiple steps, from data collection to visualization. Here’s a structured approach:
1️⃣ Define the Problem Statement
Identify a specific problem or opportunity, such as:
- Predicting patient readmissions
- Optimizing inventory management for medical supplies
- Reducing operational costs through predictive maintenance
2️⃣ Collect and Prepare ERP Data
Extract relevant data from your ERP system, including:
- Patient records
- Inventory and supply chain data
- Financial and operational data
🔎 Ensure your data is clean, accurate, and consistent before analysis.
3️⃣ Choose the Right Data Science Techniques
Depending on the problem, use:
- Predictive Analytics — Forecast patient readmissions or medical supply demand.
- Machine Learning — Identify patterns in patient data or optimize resource allocation.
- Natural Language Processing (NLP) — Analyze doctor’s notes or patient feedback.
4️⃣ Develop and Test the Model
- Build a data science model using Python, R, or TensorFlow.
- Train the model on historical ERP data and test its accuracy.
5️⃣ Visualize the Results
- Use Tableau, Power BI, or Matplotlib to present insights clearly.
- Create dashboards highlighting key metrics like readmission rates or inventory levels.
6️⃣ Evaluate the PoC
Assess the success of the PoC based on:
- Accuracy of predictions
- Cost savings achieved
- Operational efficiency improvements
- Stakeholder feedback
📊 Real-World Use Cases for Data Science in Healthcare ERP Systems
🏥 Predictive Analytics for Patient Readmissions
Problem: High readmission rates lead to increased costs and poor patient outcomes.
Solution: Use predictive analytics to identify patients at risk of readmission based on medical history, treatment plans, and demographics.
Outcome: Reduce readmission rates by providing targeted interventions for high-risk patients.
🛠️ Inventory Optimization for Medical Supplies
Problem: Overstocking or understocking medical supplies causes waste or shortages.
Solution: Machine learning predicts demand based on historical data, seasonal trends, and patient volumes.
Outcome: Optimize inventory levels, reduce waste, and ensure timely availability of critical supplies.
🔒 Fraud Detection in Healthcare Claims
Problem: Fraudulent healthcare claims result in significant financial losses.
Solution: Use anomaly detection algorithms to spot suspicious patterns in claims data.
Outcome: Reduce fraud and enhance financial performance.
🤖 Personalized Treatment Plans
Problem: One-size-fits-all treatment plans may not work for all patients.
Solution: Machine learning analyzes patient data to recommend personalized treatment plans.
Outcome: Improved patient outcomes and satisfaction.
⚠️ Challenges and Considerations
While Data Science in Healthcare ERP Systems offers immense benefits, there are challenges to address:
📂 Data Quality — Inaccurate data can lead to misleading insights.
🔧 Integration Complexity — ERP systems must be properly integrated with data science tools.
🔒 Privacy & Security — Healthcare data is sensitive. Ensure compliance with HIPAA and GDPR.
🌟 Stakeholder Buy-In — Use a PoC to demonstrate real-world value and gain support.
📝 Conclusion: Unlocking the Full Potential of Healthcare ERP Systems
Healthcare ERP systems contain a treasure trove of data, but without the right tools, this data remains underutilized. Data Science in Healthcare ERP Systems helps organizations improve patient outcomes, optimize operations, and cut costs 💸.
By following the steps outlined in this blog, you can build a successful Data Science PoC for your healthcare ERP system and harness the power of data-driven decision-making ✨.
👥 Call-to-Action (CTA)
🌟 Ready to unlock hidden insights in your healthcare ERP system? Contact us today to build a Data Science Proof of Concept (PoC) tailored to your organization’s needs. Let’s work together to drive innovation and improve outcomes in healthcare!